Dr. Jason Rambach, coordinator of the EU Horizon Project HumanTech co-organized a workshop on “AI and Robotics in Construction” at the European Robotics Forum 2023 in Odense, Denmark (March 14th to 16th, 2023) in cooperation with the construction Robotics projects Beeyonders and RobetArme.
From the project HumanTech, Jason Rambach presented an overview of the project objectives as well as insights into the results achieved by Month 9 of the project. Patrick Roth from the partner Implenia, presented the perspective and challenges of the construction industry on the use of Robotics and AI in construction sites, while the project partners Dr. Bharath Sankaran (Naska.AI) and Dr. Gabor Sziebig (SINTEF) participated in a panel session discussing the future of Robotics in construction.
Workshop schedule: https://erf2023.sdu.dk/timetable/event/ai-and-robotics-in-construction/
HumanTech project: https://humantech-horizon.eu/
Contact: Dr. Jason Rambach
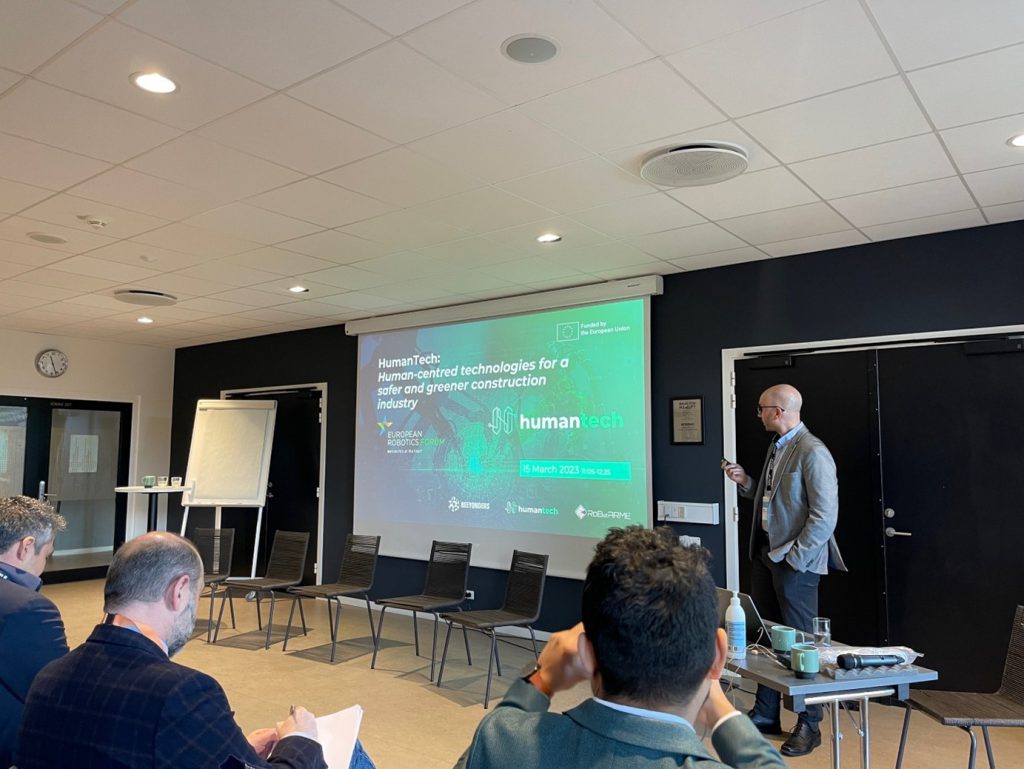