DFKI Augmented Vision is collaborating with Stellantis on the topic of Radar-Camera Fusion for Automotive Object Detection using Deep Learning. Recently, two new publications were accepted to the GCPR 2023 and EUSIPCO 2023 conferences.
The 2 new publications are:
1. Cross-Dataset Experimental Study of Radar-Camera Fusion in Bird’s-Eye View, Proceedings of the 31st. European Signal Processing Conference (EUSIPCO-2023), September 4-8, Helsinki, Finland, IEEE, 2023.
Lukas Stefan Stäcker, Philipp Heidenreich, Jason Rambach, Didier Stricker
This paper investigates the influence of the training dataset and transfer learning on camera-radar fusion approaches, showing that while the camera branch needs large and diverse training data, the radar branch benefits more from a high-performance radar.
Cross-Dataset Experimental Study of Radar-Camera Fusion in Bird’s-Eye View
2. RC-BEVFusion: A Plug-In Module for Radar-Camera Bird’s Eye View Feature Fusion, Proceedings of. Annual Symposium of the German Association for Pattern Recognition (DAGM-2023), September 19-22, Heidelberg, BW, Germany, DAGM, 9/2023.
Lukas Stefan Stäcker, Shashank Mishra, Philipp Heidenreich, Jason Rambach, Didier Stricker
This paper introduces a new Bird’s Eye view fusion network architecture for camera-radar fusion for 3D object detection that performs favorably on the NuScenes dataset benchmark.
RC-BEVFusion: A Plug-In Module for Radar-Camera Bird’s Eye View Feature Fusion
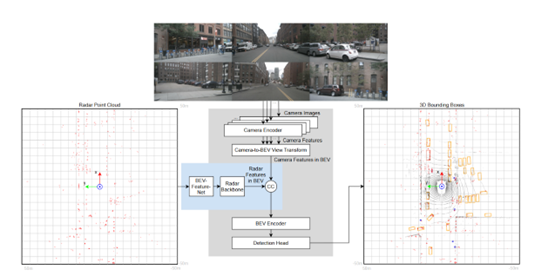
Contacts: Dr. Jason Rambach