News
Our former colleagues took the 1st (ioxp) and 3rd (3Digify) place in the 4th Südwest-Pitch organized by Startup Westpfalz and SAR.FACTORY.
For more details visit Südwest-Pitch.
We are happy to announce that two papers of our research group were accepted for presentation in different conferences.
Paper #1: Gravitational Approach for Point Set Registration by at CVPR 2016 from June26th – July 1st in Las Vegas.
CVPR is the premier annual computer vision event comprising the main conference and several co-located workshops and short courses. With its high quality and low cost, it provides an exceptional value for students, academics and industry researchers.
Paper #2: Joint Pre-Alignment and Robust Rigid Point Set Registration by Vladislav Golyanik, Bertram Taetz, Didier Stricker at ICIP 2016 from September 25th -28th in Phoenix.
ICIP is the world’s largest and most comprehensive technical conference focused on image and video processing and computer vision. The conference will feature world-class speakers, tutorials, exhibits, and a vision technology showcase.
Our avatar reconstruction pipeline is currently being tested and helping to develop new therapies for schizophrenic, autism and other social phobias.
The European Community Research and Development Information Service has a news piece on AlterEgo:
http://cordis.europa.eu/news/rcn/124883_en.html
Best Paper Award at WACV 2016
For their paper “Occlusion-Aware Video Registration for Highly Non-Rigid Objects”, Bertram Taetz, Gabriele Bleser, Vladislav Golyanik, and Didier Stricker have won the Best Paper Award at the Winter Conference on the Applications of Computer Vision – WACV 2016 on March 9, 2016 in Lake Placid, USA. Congratulations to the authors of the paper!
From 14 to 18 March 2016 our spin-offs will be presenting the results of the department’s research work at CeBIT 2016.
Our colleagues from ioxp will be presenting the AR Handbook in hall 6, booth B48.
If you are interested in 3D scanners for everyone, don’t miss 3Digify in hall 6, booth C17.
We are looking forward to welcoming you at our booths!
Three papers have been accepted at IEEE Winter Conference on Applications of Computer Vision (WACV 2016):
CoRBS: Comprehensive RGB-D Benchmark for SLAM using Kinect v2
Oliver Wasenmüller, Marcel Meyer, Didier Stricker
Extended Coherent Point Drift Algorithm with Correspondence Priors and Optimal Subsampling
Vladislav Golyanik, Bertram Taetz, Gerd Reis, Didier Stricker
Occlusion-Aware Video Registration for Highly Non-Rigid Objects
Bertram Taetz, Gabriele Bleser, Vladislav Golyanik, Didier Stricker
The WACV is one of the most renowned conferences covering all areas of Computer Vision.
It will be held March 7-9, 2016 at the Crowne Plaza resort in Lake Placid, NY, USA.
The Augmented Vision department and its partners are presenting three projects at the ICT 2015.
Come and visit:
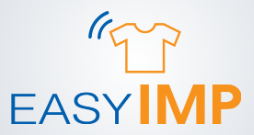
Location: Off-site Area (Praça do Comércio, Lisbon)
Exhibition date: 18 – 22 October 2015
Project homepage: http://www.easy-imp.eu/
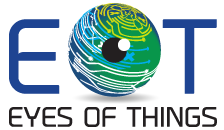
Location: Transform Area
Booth: T26
Exhibition date: 20 – 22 October 2015
Project homepage: http://eyesofthings.eu
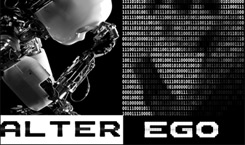
Location: Innovate Area
Booth: i33
Exhibition date: 20 – 22 October 2015
Project Homepage: www.euromov.eu/alterego
A paper was accepted as oral presentation at the International Conference on Computer Vision (ICCV) 2015:
Christian Bailer, Bertram Taetz, Didier Stricker
Flow Fields: Dense Correspondence Fields for Highly Accurate Large Displacement Optical Flow Estimation
ICCV is one of the premier conferences for researchers in the field of computer vision.
The conference will be held from December 11th to September 18th in Santiago (Chile).
We are delighted to announce the winning of smartDoc competition (challenge 2) as part of the competitions in ICDAR 2015 Conference.
The goal of the competition is to extract the textual content from document images which are captured by mobile phones. The images are taken under varying conditions to provide a challenging input (full description of the challenge).
The method is based on “Combining Clustering and Classfication Results” (CCC); initially the background color is used to first detect and dewarp the document. The image is then binarized to extract lines, words and subwords. Those are then clustered incrementally across all the corpus. A 1D LSTM is trained on both sharp and blurry gray-scale text-lines for recognizing subwords. Clusters of subwords are labeled by majority voting.
Each year an expert jury from the University of Kaiserslautern is nominating outstanding doctoral theses for several academic subjects together with the Kreissparkasse Kaiserslautern..
We congratulate Dr.-Ing. Petersen on winning this award for Computer Science.
This award was granted for his doctoral thesis that deals with the understanding of manual workflows from video examples. An important application of his research work is the automatic generation of interactive Augmented Reality manuals from the observation of a reference performance.
Links:
Abstract doctoral thesis:
Workflow knowledge comprises both explicit, verbalizable knowledge and implicit knowledge, which is acquired through practice. Learning a complex workflow therefore benefits from training with a permanent corrective. Augmented Reality manuals that display instructive step-by-step information directly into the user’s field of view provide an intuitive and provably effective learning environment. However, their creation process is rather work intensive and current technological approaches lead to insufficient interactivity with the user. In this thesis we present a comprehensive technical approach to algorithmically analyze manual workflows from video examples and use the acquired information to teach explicit and implicit workflow knowledge using Augmented Reality. The technical realization starts with unsupervised segmentation of single work steps and their categorization into a coarse taxonomy. Thereafter, we analyze the single steps for their modalities using a hand and finger tracking approach optimized for this particular application. Using explicit, work step specific generalization we are able to compensate for morphological differences among different users and thus to reduce the need for large amounts of training data. To render this information communicable, i.e., understandable by a different person, we present the further processed data using Augmented Reality as an interactive tutoring system. The resulting system allows for fully or semi-automatic creation of Augmented Reality (AR-) manuals from video examples as well as their context-driven presentation in AR. The method is able to extract and to teach procedural, implicit workflow knowledge from given video examples. In an extensive evaluation, we demonstrate the applicability of all proposed technical components for the given task.
Prof. Didier Stricker gave a key impulse talk about „Technologiy and Innovation“ in the area of Digital Technology at the MIT:Futura. The event analyzed barriers for digitalization, creation of start-ups and large capital investment in Germany. Investors, founders and European Commissar for Digitalization – Mr Oettinger – discussed current situation and possible changes for speeding-up technological changes at economical and societal level.
The new spin-off of the department Augmented Vision of DFKI won the prestigious start-ups ICT price of the Ministry of Economy.
The team of 4 formers members of the department were congratulated at the Cebit fair 2015.
More on: www.ioxp.de
The new technology in the area of 3D scanning has been presented for the first time at the Cebit.
The big success confirmed the relevance of the approach and need for such solution.
Please register on: www.3digify.com
…is the newly started European project at AV.
The kick-off meeting was held on January 27th 2015 at the University of Castilla-La Mancha.
Read also the press release on the Kick-off meeting.
If you are interested in the project’s goals and objectives, partners and the idea behind it, please check also the project website http://eyesofthings.eu
… the WearHEALTH team for their success being one of the eight winners of the prestigious Young Researchers Competition called by the German Federal Ministry of Education and Research (BMBF) on the field of “interdisciplinary competence development in the research field human-machine interaction for demographic change”.
If you are curious and would like to know who or what is WearHEALTH .
Positions in the area of sensor networks, sensor fusion, motion tracking and computer vision / augmented Reality are available. Please refer to the following links for more details.
- Researcher Position for On-Body Sensor Network, Statistical Filtering
- Software Engineer in the area of Image Processing / Computer Vision
- Software Developer Position in the area of “Smart Sensor Networks”
- Wissenschaftliche Mitarbeiterin / Wissenschaftlicher Mitarbeiter (Teilzeit)
- PhD position Inertial MoCap and Motion Interpretation
- Researcher Position in Image Processing, esp. Object Recognition and Tracking
Two publications were accepted at the European Conference on Computer Vision (ECCV) 2014. ECCV is one of the top conferences for researchers in the field of computer vision.
Christian Bailer, Alain Pagani, Didier Stricker
A Superior Tracking Approach: Building a strong Tracker through Fusion
Tobias Nöll, Johannes Köhler, Didier Stricker
Robust and Accurate Non-Parametric Estimation of Reflectance using Basis Decomposition and Correction Functions
ECCV 2014 takes place for the 13th time and will be held from September 6th to September 12th in Zürich (Switzerland).
Alain Pagani hat am 13.06.2014 seine Dissertation mit der wissenschaftlichen Aussprache erfolgreich abgeschlossen.
Seine Doktorarbeit trägt den Titel „Reality models for efficient registration in Augmented Reality“. In seiner Arbeit befasste sich Alain Pagani mit mathematischen Modellen realer Objekte oder Szenen, um das Problem der Kameraregistrierung im Forschungsfeld Erweiterte Realität zu behandeln. Die vorgeschlagenen bildbasierte Verfahren ermöglichen eine effiziente Kamerapose-Berechnung in unterschiedlichen Szenarien und lösen somit eine grundliegende Herausforderung der erweiterten Realität.
Gutachter der Arbeit sind Prof. Dr. Didier Stricker und Prof. Dr. Michael Felsberg (Linköping University).
Wir gratulieren Alain Pagani herzlich zu diesem Erfolg!