SALT: A Semi-automatic Labeling Tool for RGB-D Video Sequences
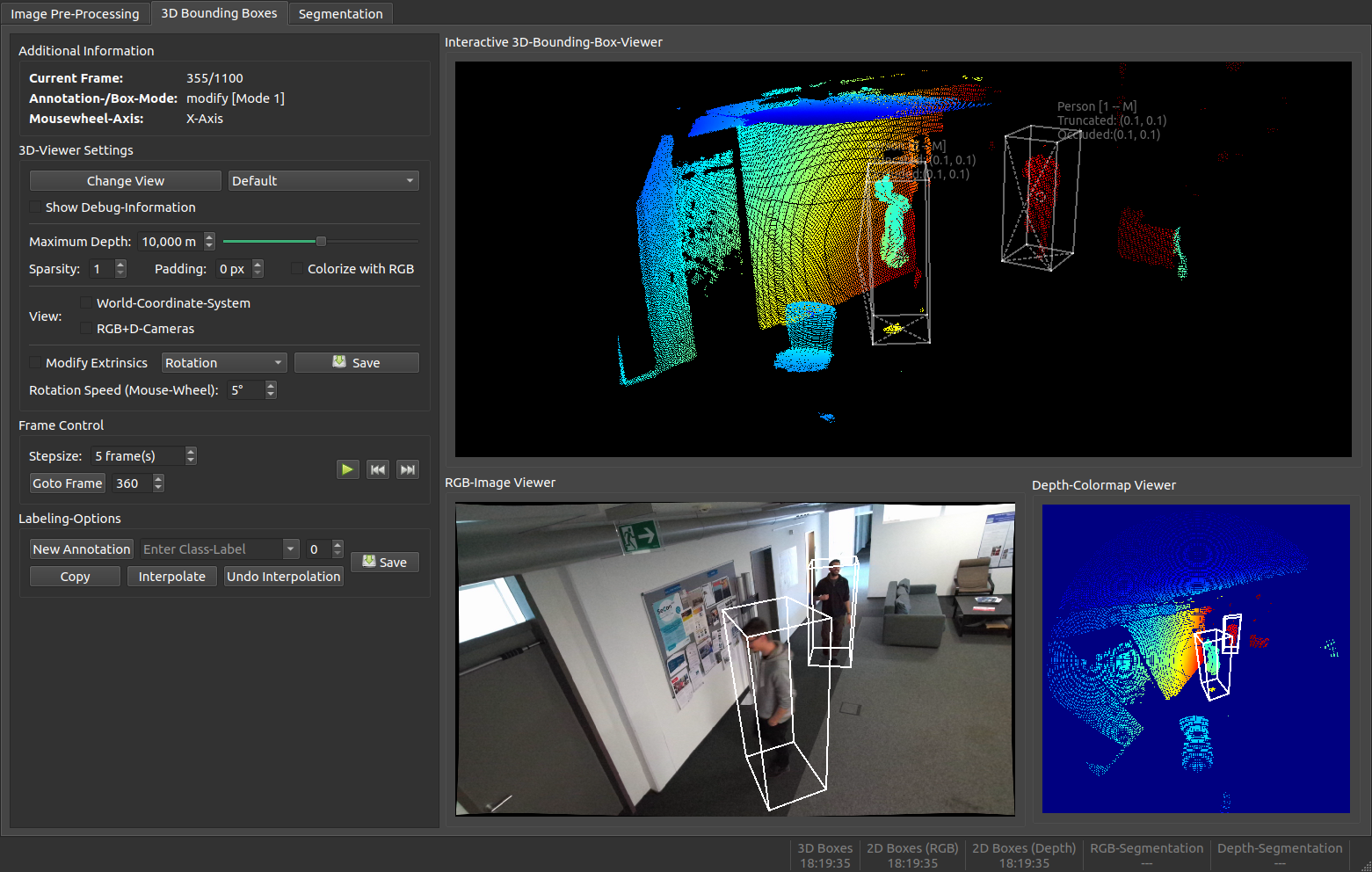
SALT: A Semi-automatic Labeling Tool for RGB-D Video Sequences
Proceedings of the 16th International Joint Conference on Computer Vision, Imaging and Computer Graphics Theory and Applications (VISIGRAPP-2021). International Conference on Computer Vision Theory and Applications (VISAPP-2021) 16th International Conference on Computer Vision Theory and Applications February 8-10 Online (due to COVID-19) ISBN TBA SCITEPRESS 2021 .
- Abstract:
-
Large labeled data sets are one of the essential basics of modern deep learning techniques. Therefore, there is an increasing need for tools that allow to label large amounts of data as intuitively as possible. In this paper, we introduce SALT, a tool to semi-automatically annotate RGB-D video sequences to generate 3D bounding boxes for full six Degrees of Freedom (DoF) object poses, as well as pixel-level instance segmentation masks for both RGB and depth. Besides bounding box propagation through various interpolation techniques, as well as algorithmically guided instance segmentation, our pipeline also provides built-in pre-processing functionalities to facilitate the data set creation process. By making full use of SALT, annotation time can be reduced by a factor of up to 33.95 for bounding box creation and 8.55 for RGB segmentation without compromising the quality of the automatically generated ground truth.
Reference:
@inproceedings{stumpf2021salt, author={Dennis Stumpf and Stephan Krau{\"ss} and Gerd Reis and Oliver Wasenm{\"u}ller and Didier Stricker}, title={SALT: A Semi-automatic Labeling Tool for RGB-D Video Sequences}, booktitle={Proceedings of the 16th International Joint Conference on Computer Vision, Imaging and Computer Graphics Theory and Applications - Volume 4 VISAPP: VISAPP}, year={2021}, pages={595-603}, publisher={SciTePress}, organization={INSTICC}, doi={10.5220/0010303005950603}, isbn={978-989-758-488-6}, }