We are very happy to announce that our paper “HandVoxNet++: 3D Hand Shape and Pose Estimation using Voxel-Based Neural Networks” has been accepted for publication in the renowned journal “IEEE Transactions on Pattern Analysis and Machine Intelligence“.
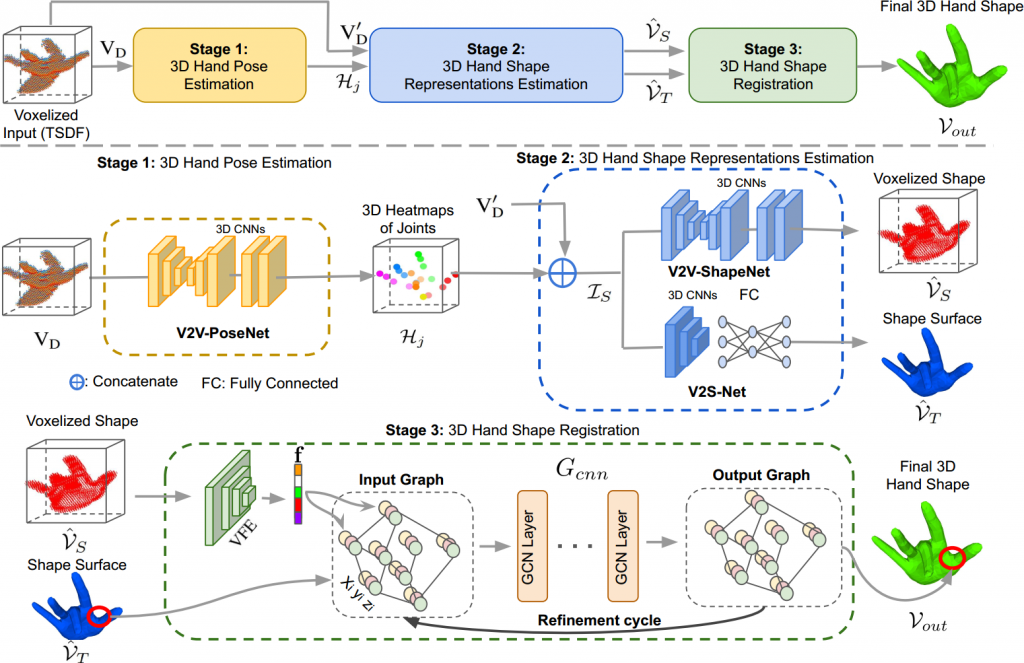
We introduce a new HandVoxNet++ method for 3D hand shape and pose reconstruction from a single depth map, which establishes an effective inter-link between hand pose and shape estimations using 3D and graph convolutions. The input to our network (i.e., HandVoxNet++) is a 3D voxelized depth map based on the truncated signed distance function (TSDF), and it relies two hand shape representations. The first one is the 3D voxelized grid of hand shape, which does not preserve the mesh topology and which is the most accurate representation. The second representation is the hand surface that preserves the mesh topology. We combine the advantages of both representations by aligning the hand surface to the voxelized hand shape either with a new neural Graph-Convolutions-based Mesh Registration (GCN-MeshReg) or classical segment-wise Non-Rigid Gravitational Approach (NRGA++) which does not rely on training data. In this journal extension of our previous approach presented at CVPR 2020, we gain 41.09% and 13.7% higher shape alignment accuracy on SynHand5M and HANDS19 datasets, respectively. Our results indicate that the one-to-one mapping between voxelized depth map, voxelized shape and 3D heatmaps of joints is essential for an accurate hand shape and pose recovery.
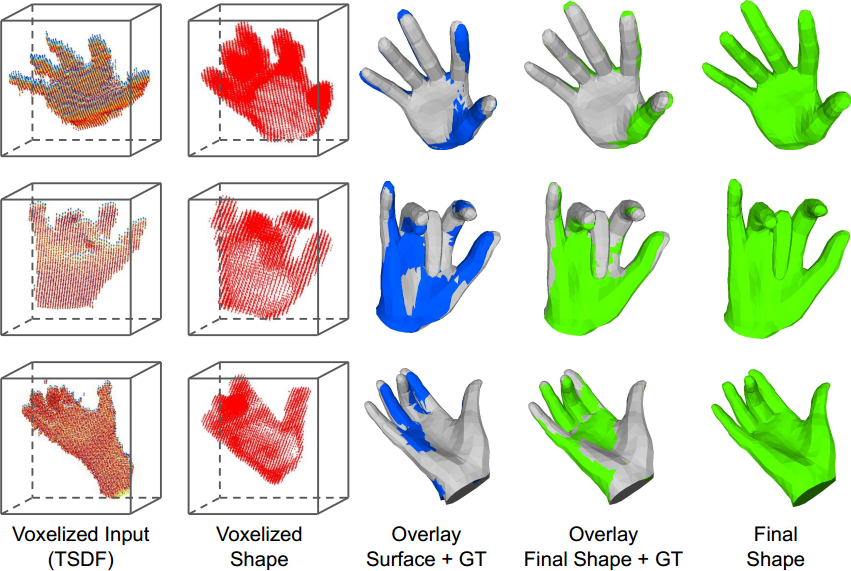